Evaluating Urban Transportation Policies
Traffic congestion poses a significant challenge in urban centers, especially in fast-growing emerging economies where rapid urbanization and increased travel demand have outpaced road infrastructure and regulations. Longer travel times and worsened air quality resulting from congestion hinder mobility and urban development while reducing the overall quality of life. In the 2018 TomTom Traffic Index, which is based on real-time GPS traffic data from 403 cities in 56 countries, the 10 most congested cities were all in developing and emerging economies. In these cities, commuters spent over 200 hours of extra travel time per year relative to when transport was flowing freely.
Local governments have implemented a range of policies to address traffic congestion, targeting both the demand and supply sides of road infrastructure. On the demand side, policies encompass command-and-control style driving restrictions, vehicle purchase quota systems, and market-based congestion pricing. On the supply side, efforts have been made to expand public transit options and to enhance road capacity.
This summary describes our research on the impact of various urban transportation policies aimed at alleviating traffic congestion and air pollution. We focus on measuring crucial quantities, including the marginal external cost of traffic congestion, and evaluating different policies in terms of both efficiency and equity within an integrated framework. Much of our analysis focuses on Beijing. With a population of over 21 million, the city has consistently ranked among the most congested in the world. Its municipal government has implemented aggressive demand-side and supply-side policies over the past 15 years, making it an ideal setting for studying urban transportation policies.
Estimating the Marginal External Cost of Congestion
Economic theory indicates that the optimal congestion charge is equal to the marginal external cost of congestion (MECC) at the socially optimal level of traffic. The MECC critically hinges on the incremental effect of traffic density on traffic speed: how much an additional vehicle on the road slows down the traffic. Empirical estimation of the density-speed relationship is subject to the endogeneity challenge, as speed and density affect each other and both are equilibrium outcomes influenced by idiosyncrasies. Our study provides, to our knowledge, the first causal estimate of the density-speed relationship by leveraging plausibly exogenous variations in traffic introduced by Beijing’s driving restriction policy.1
There are six circumferential or “ring” roads around central Beijing. Government policy prohibits certain vehicles from driving within the fifth ring road from 7 am to 8 pm during workdays. There is a predetermined rotation schedule based on the last digit of a vehicle’s license plate. There are days for numbers 1 and 6, 2 and 7, 3 and 8, 4 and 9, and 5 and 0. Due to the nonuniform distribution of the last digit of license plate numbers, the policy exogenously shifts the number of vehicles on the road. Notably, vehicles with license plates ending in the number 4 constitute only about 2 percent of all vehicles due to Chinese cultural aversion to the number 4. Consequently, on days when vehicles with license plates ending in 4 and 9 are restricted, there are more vehicles on the road, leading to heightened congestion compared to other days. This variation in traffic speed and density as shown in Figure 1 is used to establish the causal relationship between traffic speed and density.
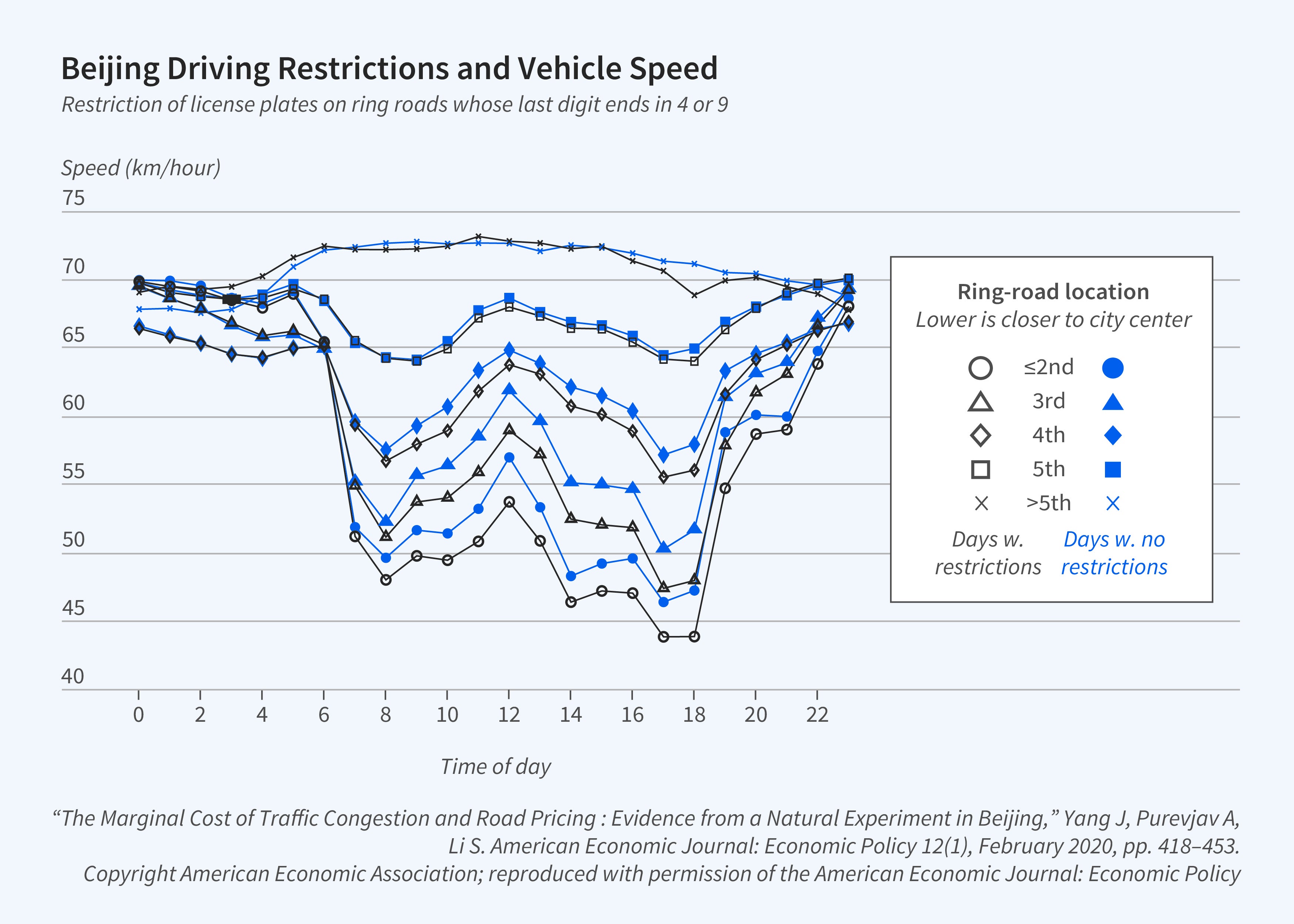
Our analysis, utilizing a year’s worth of hourly traffic data from about 1,500 monitors in Beijing, reveals that addressing endogeneity in the relationship between speed and density results in a 60 percent increase in the estimate of the MECC compared to that obtained through an ordinary least squares regression. Therefore, relying on the latter would induce a significant downward bias in optimal congestion charges. Additionally, the MECC exhibits notable heterogeneity over time and particularly across different locations. Our analysis demonstrates that implementing time-varying and location-specific congestion charges could lead to substantial congestion reduction, welfare gains, and increased government revenue.
A Unified Framework for Policy Comparison
Our studies are part of a large literature that examines the effects of transportation policies on outcomes such as vehicle ownership, travel mode choices, traffic congestion, air pollution, housing prices, and job access. Our analysis of vehicle quota systems suggests that while a lottery system is more equitable and effective than an auction in reducing automobile externalities, this advantage is offset by a significant cost from misallocation.2 Studying the opening of 14 new subway lines during 2008–16 in Beijing, we found that subway expansions improved air quality, but that the resulting health benefit was small relative to the construction and operating costs. Hence, the cost of subway expansion would need to be justified by traffic congestion relief and other economy-wide impacts.3 With respect to driving restrictions, we find that the policy in Beijing steepened the housing bid-rent curve, led to a higher premium for properties closer to subway stations, and changed the spatial distribution of households around subway lines.4
Empirical studies that evaluate and compare different policies within a unified framework are scarce. To address this gap, we have developed an equilibrium model of residential sorting that allows us to compare the efficiency and equity impacts of various transportation policies.5
In the model, households choose a residence based on the job locations of their working members. A key consideration is ease of commute for each working member. The ease-of-commute measure is derived from a model of travel mode choices and crucially depends on traffic congestion, which varies across locations and results from all households’ travel choices and residential locations. Our explicit modeling of the travel mode choices to derive the ease-of-commute measure provides a microfoundation for the linkage of the housing market and the transportation sector. This modeling choice represents an important departure from the literature which typically uses distance to the central business district to capture the ease of the commute without endogenizing congestion.
In the housing market, choices of individual households aggregate to total housing demand and house prices adjust to equalize demand and supply. In the transportation sector, the equilibrium congestion level and hence driving speed are jointly determined by the driving demand of all individuals and road capacity. These two markets interact in two dimensions: the spatial locations of households affect the distance of work commutes and the choice of travel mode, hence congestion and driving speeds. At the same time, the level of traffic congestion affects the attractiveness of residential locations through ease-of-commute considerations; the attractiveness of different locations, in turn, feeds back to shape the spatial distribution of households.
The model premises include two sets of preferences that govern household choices: preference parameters for housing attributes including the ease-of-commute measure, and preference parameters for travel mode attributes such as travel time and travel cost. With these underlying parameters estimated, the model allows us to conduct counterfactual simulations to predict new equilibrium outcomes for house prices, congestion, and welfare under different policy scenarios.
We rely on two rich datasets for estimation. One is the Beijing Household Travel Survey, a large representative survey that records households’ home and work locations, trips made in a 24-hour window, and other demographic and transportation-related information. Based on application programming interface (API) requests from online mapping service and geographic information system software, we compile the commuting route, distance, travel time, and cost for each travel mode of all home-to-work trips. The other dataset contains housing transactions from a major government-run mortgage program and provides a large representative sample of Beijing homebuyers. Critically for our analysis, the housing data report not only the home location but also the work locations of household members. We then construct over 13 million hypothetical work-commute and travel-mode combinations for all properties in each homebuyer’s choice set, using the same procedure as in the travel survey.
Our estimation follows a two-step procedure. The first recovers heterogeneous preferences for travel times and monetary costs — and thereby the value of time — based on the travel data. We then utilize the estimated parameters from this step and household members’ work locations to construct the ease-of-commute measure separately for each commuter in the household and for all properties in a household’s choice set. These variables are included as household-property-specific attributes in the housing demand estimation in the second step, which recovers preferences for housing attributes, including the preference for ease of commute, based on the housing transaction data.
We simulate equilibrium residential sorting and transportation outcomes under different policies: driving restrictions, subway expansion, distance-based congestion pricing, as well as combinations of the three such as subway expansion plus driving restrictions and subway expansion plus congestion pricing. To facilitate comparison, the congestion charge is chosen to achieve the same level of congestion reduction as driving restrictions, though our model also yields estimates of the optimal congestion charge.
Our policy simulations provide four important findings. First, different transportation policies exhibit distinct efficiency properties (Figure 2). While driving restrictions and congestion pricing achieve the same level of congestion reduction by design, congestion pricing improves welfare but driving restrictions reduce it because of the large distortion in travel mode choices. Beijing’s rapid subway expansion increased aggregate welfare, despite the fact that it achieved only a modest congestion reduction. Congestion pricing and subway expansion in tandem deliver the largest improvement to traffic speed and net welfare gain — equivalent to 3 percent of average household income. In addition, revenue from congestion pricing could fully finance capital and operating costs of subway expansion, eliminating the need to resort to distortionary taxes.
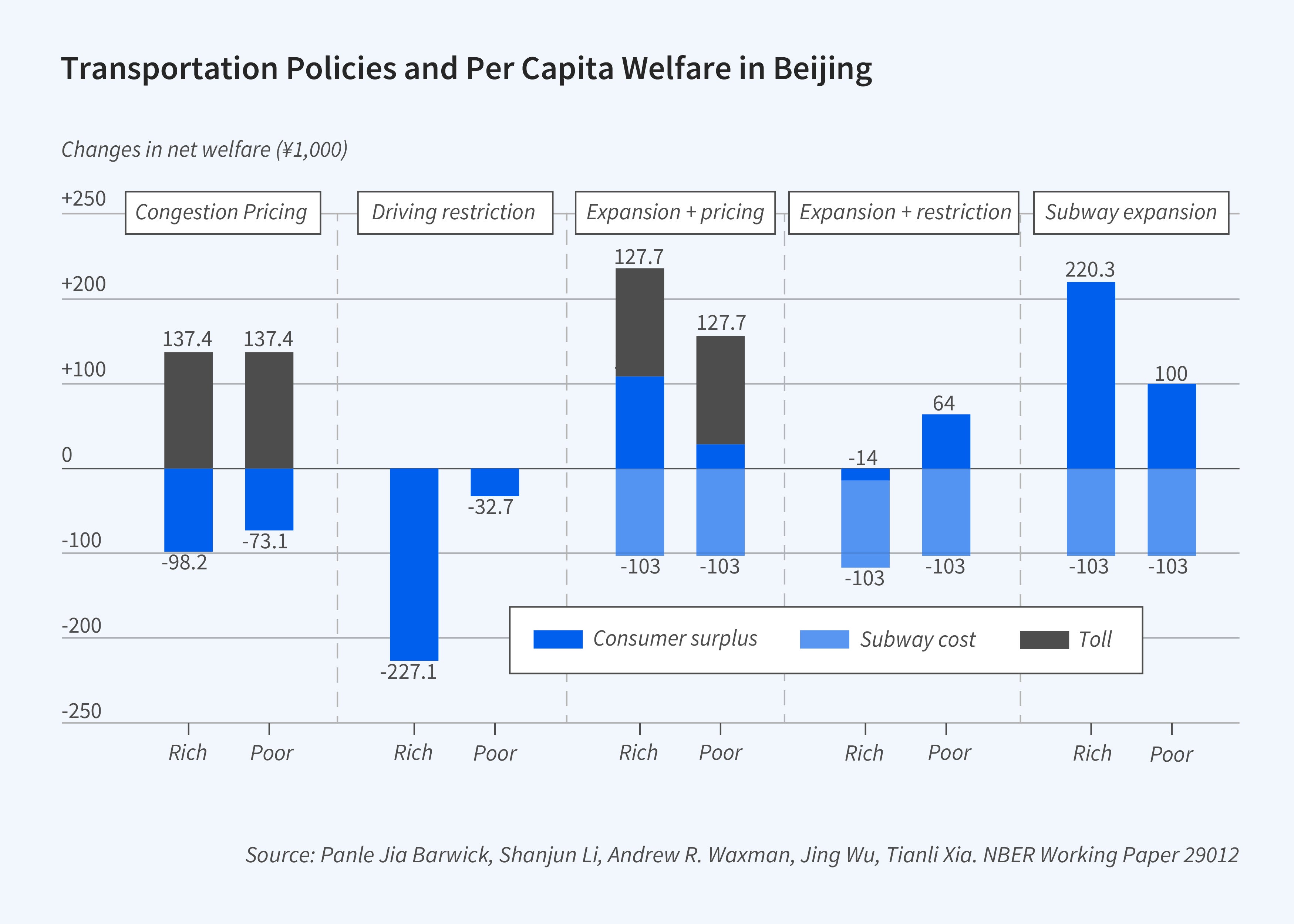
Second, policies differ in terms of distributional consequences. Without revenue recycling, congestion pricing is regressive, which poses a substantial obstacle to its practical implementation. In contrast, driving restrictions and particularly subway expansion are progressive, which likely contributes to their greater adoption in practice. However, it is worth noting that with appropriate revenue recycling, congestion pricing can be welfare enhancing for low-income households, thereby addressing distributional concerns.
Third, although all three policies help alleviate congestion, they have distinct and even contrasting effects on the spatial distribution of residential areas and equilibrium housing prices (Figure 3). Distance-based congestion pricing creates strong incentives for both high- and low-income commuters to relocate closer to their workplaces. In contrast, subway expansion increases the spatial separation between residential and job locations by dispersing households from the city center toward suburban areas and locations near new subway stations.
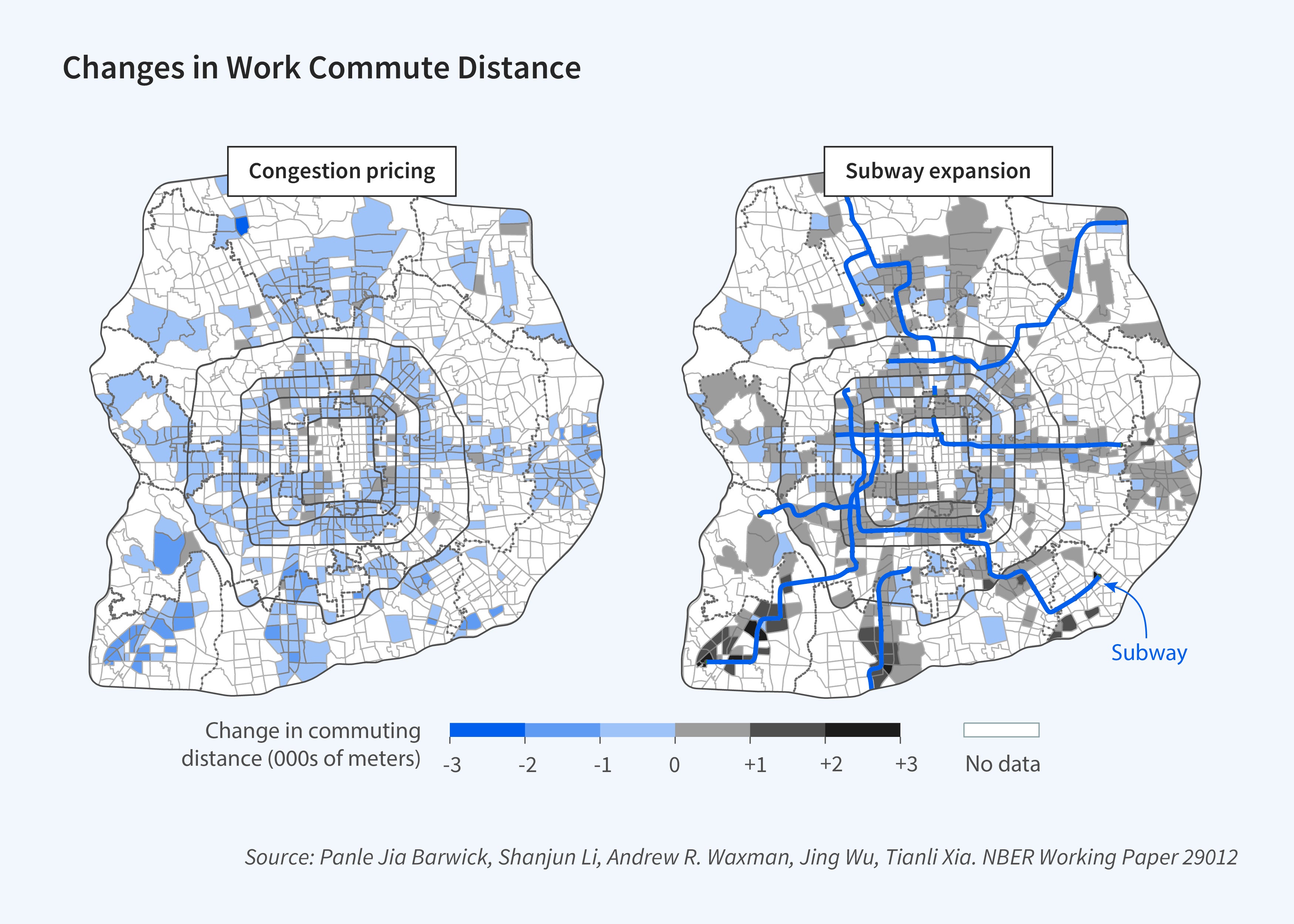
Finally, residential sorting can either bolster or undermine the effectiveness of transportation policies aimed at reducing congestion. Sorting reinforces the efficiency of congestion pricing as households, particularly those with lengthy commutes, are motivated to reside closer to their workplaces and reduce driving. This especially amplifies the welfare benefits of congestion pricing for high-income households. Conversely, sorting in response to subway expansion results in increased spatial separation between residential and work areas, diminishing both the congestion-reduction effect and the welfare gains derived from this infrastructure investment.
Additional simulations reveal that our aggregate welfare findings are sensitive to whether we endogenize traffic congestion, and that excluding preference heterogeneity induces a substantial change in the welfare estimates. These findings underscore the advantages of our equilibrium sorting model as a unified framework for policy analysis. The model effectively captures diverse adjustment mechanisms while allowing for general equilibrium effects and preference heterogeneity.
Endnotes
“The Marginal Cost of Traffic Congestion and Road Pricing: Evidence from a Natural Experiment in Beijing,” Yang J, Purevjav A, Li S. American Economic Journal: Economic Policy 12(1), February 2020, pp. 418–453.
“Better Lucky Than Rich? Welfare Analysis of Automobile Licence Allocations in Beijing and Shanghai,” Li S. The Review of Economic Studies 85(4), October 2018, pp. 2389–2428.
“Does Subway Expansion Improve Air Quality?” Li S, Liu Y, Purevjav A, Yang L. Journal of Environmental Economics and Management 96(C), July 2019, pp. 213–235.
“The Impact of Road Rationing on Housing Demand and Sorting,” Jerch R, Barwick P, Li S, Wu J. SSRN, March 2021.
“Efficiency and Equity Impacts of Urban Transportation Policies with Equilibrium Sorting,” Barwick P, Li S, Waxman A, Wu J, Xia T. NBER Working Paper 29012, February 2022.