A General Synthetic Control Framework of Estimation and Inference
Over the past decade, synthetic control estimators have found wide applicability in empirical research in economics and the social sciences as tools to evaluate the effects of public policies and other interventions or events of interests. For each unit (e.g., city, state, or region) affected by the intervention of interest, a synthetic control is a combination on unaffected units chosen to resemble the characteristics of the treated units before the intervention. Synthetic controls are used to estimate the outcomes that affected units would have attained in the absence of the intervention of interest, providing a benchmark against which researchers can evaluate policy impacts. The synthetic control method was originally developed for cases with one or a small number of aggregate units affected by the policy of interest. However, the applied literature has surpassed the methodological contributions in this area. In particular, many current applications focus on settings with a large number of affected units. As a result, additional methodological research is needed to guide the empirical implementation of the method. This research will provide empirical researchers with a robust and general framework for estimation and inference with synthetic controls as well as freely available software with which to implement it.
When disaggregated data are available, constructing separate synthetic controls for each treated unit helps avoid interpolation biases. However, the problem of finding a synthetic control that best reproduces the characteristics of a treated unit may not have a unique solution, and multiplicity of solutions is a particularly daunting challenge in settings with many treated units. This research will provide a generalized synthetic control framework for estimation and inference. The framework builds on synthetic controls and introduces a penalization parameter that trades off pairwise matching discrepancies with respect to the characteristics of each unit in the synthetic control against matching discrepancies with respect to the characteristics of the synthetic control unit as a whole. It can be shown that, as long as the penalization parameter is positive, the generalized synthetic control estimator is unique and sparse. The investigators propose data driven choices for the penalization parameter, as well as inferential methods for synthetic controls in settings with many treated units.
Investigator
Supported by the National Science Foundation grant #1756692
Related
Topics
Programs
More from NBER
In addition to working papers, the NBER disseminates affiliates’ latest findings through a range of free periodicals — the NBER Reporter, the NBER Digest, the Bulletin on Retirement and Disability, the Bulletin on Health, and the Bulletin on Entrepreneurship — as well as online conference reports, video lectures, and interviews.
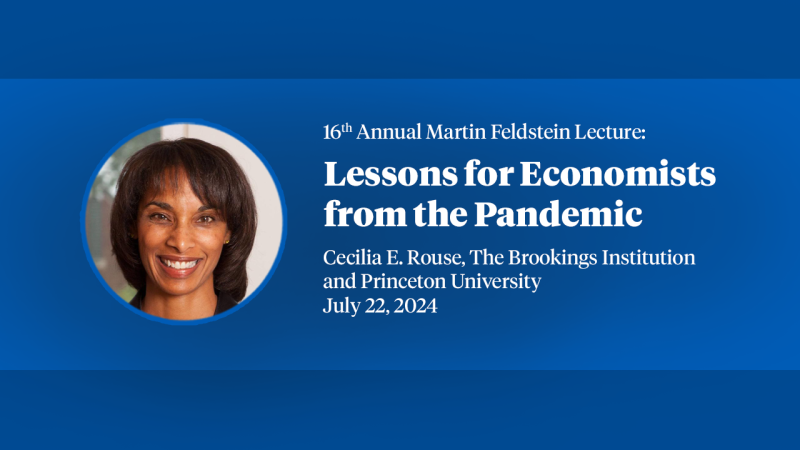
- Feldstein Lecture
- Presenter: Cecilia E. Rouse
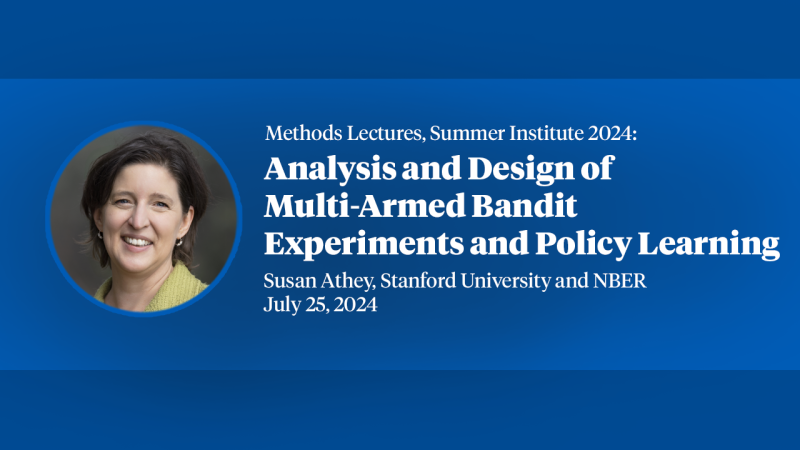
- Methods Lectures
- Presenter: Susan Athey