Artificial Intelligence and Behavioral Economics
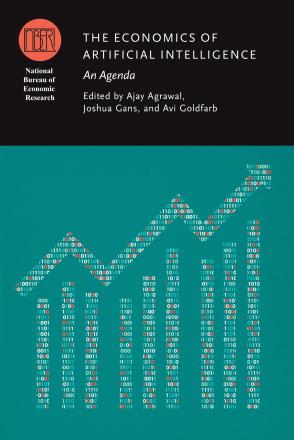
An important strand of judgment and decision research in the 1970s and 1980s, which influenced behavioral economics mostly indirectly, documented the fact that simple statistical models often predict better than experts do (beginning with Meehl 1954). This chapter revisits this phenomenon and connects it to modern machine learning (ML) debates. One view is that subjective judgment exhibits properties of “unregularized” ML which overfits, because human judgment does not naturally penalize overfitting. I also discuss how ML techniques, in large data sets, can help discover different “behavioral types” which correspond to, or extend, heterogeneous types hypothesized previously. ML applications in practice, such as recommender systems, also connect to behavioral concepts of how limited attention and preference assembly create opportunities to either benefit or harm consumers.