Bayesian Variable Selection for Nowcasting Economic Time Series
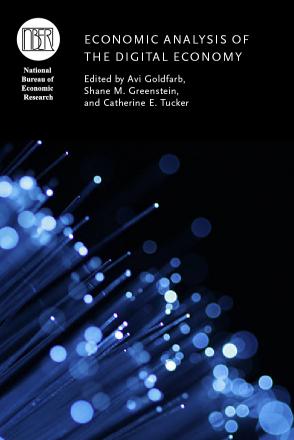
We consider the problem of short-term time series forecasting (nowcasting) when there are more possible predictors than observations. The motivating example is the use of Google Trends search engine query data as a contemporaneous predictor of economic indicators. Our preferred approach combines three Bayesian techniques: Kalman filtering, spike-and-slab regression, and model averaging. The Kalman filter can be used to control for time series feature, such as seasonality and trend; the regression can be used to incorporate predictors such as search engine queries; and model averaging can be used to reduce the danger of overfitting. Overall the Bayesian approach allows a flexible way to incorporate prior knowledge, both subjective and objective, into the estimation procedure. We illustrate this approach using search engine query data as predictors for consumer sentiment and gun sales.