Off to the Races: A Comparison of Machine Learning and Alternative Data for Predicting Economic Indicators
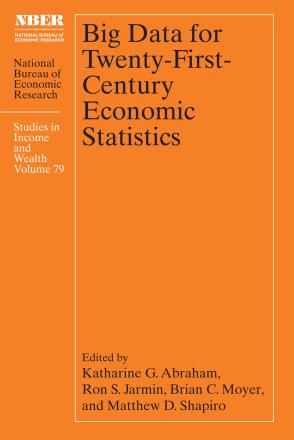
Timely alternative data sources such as credit card transactions and search query trends have become more readily available in recent years, while sophisticated machine learning (ML) techniques have enabled marked gains in predictive accuracy. These advances offer the benefit of revealing economic news earlier in the estimation cycle, reducing revisions, and improving estimate quality. But which combinations of data and ML techniques give the most accurate prediction of national economic activity? To answer this question, we conduct a prediction horse race using a one-step ahead model validation design to evaluate how each ML algorithm, data set, and variable selection method weighs on predictive accuracy. We test 73,884 model specifications, consider 1,180 variables drawn from both traditional and alternative sources, and predict 188 quarterly revenue and expenditure series for the services sector as published in the Quarterly Service Survey (QSS)—a key data set that accounts for nearly 80 percent of the revisions to Personal Consumption Expenditure for Services (PCE Services). Our results indicate that ensemble methods such as Random Forests afford the highest chance of reducing revisions. Relative to current national accounting methods, ensemble methods could reduce overall PCE revisions by 12 percent on average, with proportionally larger improvements among PCE subcomponents. While alternative data are timelier, we find evidence that traditional data such as employment and lagged dependent variables contain relatively greater signaling power than alternative data; this finding demonstrates that more data does not necessarily translate into significantly better predictions.